
Fostering Opportunities of Refugee Workers
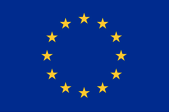
This project has received financial support from the European Union Programme for Employment and Social Innovation "EaSI" (2014- 2020)
Recent Posts
- La sfida dell’accoglienza – Article La Repubblica 1 April 2022
- FORWORK Albania – Final report 30 November 2021
- FORWORK Italy – Impact evaluation report 1 December 2021
- FORWORK Italy – Description of activities 30 November 2021
- Stories from FORWORK 25 October 2021
Nothing Found
Sorry, no posts matched your criteria